Michel Tricot (Airbyte) Fireside Chat
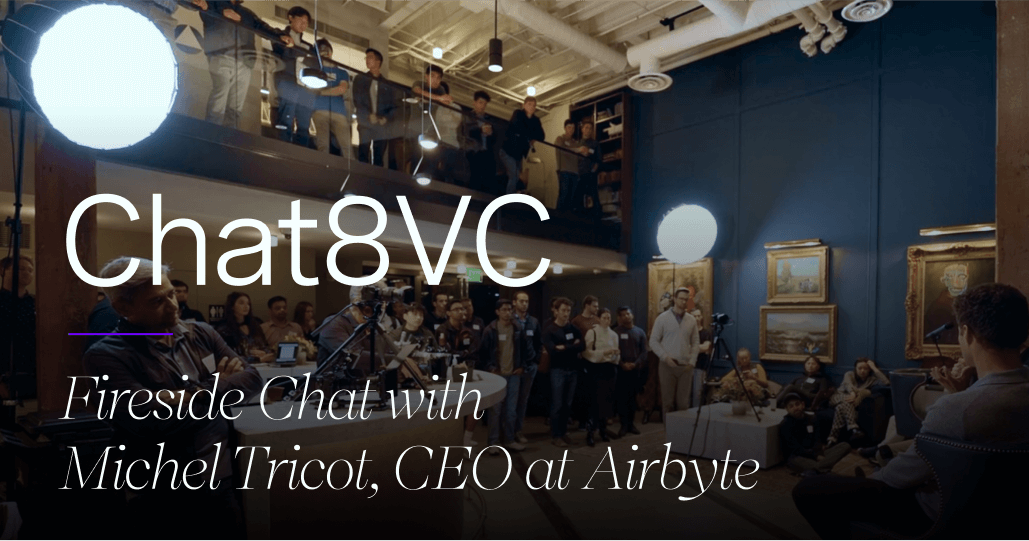
Share
We were delighted to engage in a dynamic conversation with Michel Tricot, co-founder and CEO of Airbyte, during our October Chat8VC gathering. As a reminder, Chat8VC is a monthly series hosted at our SF office where we bring together founders and early-phase operators excited about the foundation model ecosystem. We focus on having highly technical, implementation-oriented conversations and give founders and builders the opportunity to showcase what they’re working on through lightning demos and fireside chats. This can be a fun side project, related to a company they’re starting, or a high-leverage use case within a company operating at scale.
In conversation with Jack Moshkovich (8VC Principal), Michel shed light on the founding story behind Airbyte, identifying PMF and the future of ETL.
If any of these themes excite you and you’d like to chat more with our team about them or attend future Chat8VC events, please reach out to Vivek Gopalan at vivek@8vc.com and Bela Becerra at bela@8vc.com!
Michel – you and I first met in March of 2020. Back then, people thought the world was about to end. Airbyte has a very unique inception story, both around how the business was started, but also more specifically about how the business ended up being what it is today. Tell us a bit more about that.
Our story is certainly full of ups and downs. Airbyte today is often associated with our open source data infrastructure project and product. It really started, though, in July 2019, when my co-founder and I first decided to build something together. We implemented a fairly disciplined system around idea exploration and honed in on the problem space of data and went through YC in January ‘20, which was the famous COVID batch where we went from being in person to fully remote.
At the time, we were tinkering in the data integration space and focusing on how to bring more data to a marketing audience. As it felt like the world was ending in March ‘20, many companies were laying off their marketing teams at the time. So just as we were starting to have amazing conversations from January to March, we suddenly got replies over email saying, "This email address is invalid." And that was really the reality we were living through. And for us, we had actually just hired three amazing engineers for this product. Although we had an idea for a data product, we had no one to sell it to. We realized we needed to determine if we were building a product that is a good-to-have, or a need-to-have. As we were contemplating this, you actually really helped us evaluate how to best navigate the pivot.
In hindsight, there was a very funny conversation we had, which was, "Okay, we're going to pivot the company, what should we do?" One of the ideas we had was an AI-enabled assistant, which three years ago didn’t seem like a great idea. Today it has become consensus very fast, so I'm glad we picked what we picked.
At that time we gave up on the idea of building data integration for marketing, and we went back to the basics. We spent eight hours every single day – my co-founder and I – just talking and getting on the phone with people. And at the time, people had nothing to do because the pandemic had just started. They were stuck in their bedroom and they just wanted to talk to someone, and we got so many calls from April until July.
Come July, we still had not built anything, but we were narrowing down on the specific problem. At that point, we said, "Okay, we're going to change how we operate. We're going to continue to do these calls, but we're going to start building a product as well, and we're giving ourselves one month. If after one month we have no traction, we go to the next idea." So, banging your head against the wall and making sure you explore all the possible things. We built the ETL product and that was Airbyte. So we never gave up on this one. It took us a few months to build the first very alpha MVP and we released it around the end of 2020.
And for us, we did not want to build a product that already existed. We needed to have something that was a true differentiator. And if you look in the data integration space – basically how you move data from point A to point B and remove the complexity of accessing that data – there are a lot of players on the market, but none of them are solving the problem at the root. Customers always end up having to buy one product and also build on the side because the existing solutions don't have the integration extensibility. With Airbyte, we decided to build it open source because what we want is to capture teams when they're thinking about building systems internally. Open source works extremely well for capturing the builders. We released it and after just one week we had 100 users of Airbyte.
Even though the product was initially terrible, people were going above and beyond to make it work. And that was a big signal for us that we had found something.
There's a real takeaway here for everyone, which is that you really do know when you have product-market fit. Those were some of the most unbelievable conversations we had back then. It was like, "Yeah, we just launched this thing, it barely works, and we have 10,000 people using it." It made no sense. And yet, there was just so much market interest at that time for the approach that we were taking. And you spent a lot of time also in customer education, really getting the messaging correct, which I think worked really well. It was awesome.
Now, when you get that kind of pull, there exists a moment when you hit a wall in terms of too much community interest. We were fighting fires, and I think it happened really around July 2021 where we hit an inflection point in terms of adoption, and the team went to a halt in terms of our ability to develop and support the community. That's when we started to refocus our effort on just consolidating our basis and just building a more solid product.
One last thing here. I've been doing this job for five years now, and I don't think I've ever witnessed anything as impressive and as well done as how you and the rest of the team navigated those few months. At no point did anyone on the team have any doubt that you're all sticking together and that you're going to figure this out, but there's very little similarity between where you started and where you went. I think that just speaks to the importance of having empathy for the rest of the team and how are people feeling about [a pivot] and truly working with people who are actually going to have your back. It's pretty cool to see.
Yeah, definitely. The founding team knew each other from before, so that helped a lot on working together. But also, when they started, we had no product at the time, so we were very, very clear with them that the product you’re building today is most likely not going to be the product you're working on next month. And that's what happened several times. So it was really about expectation-setting.
Okay, let's fast-forward to, call it 12 months ago. Airbyte is the leading open source ETL framework in the market, and the AI craze starts happening. Just maybe for those who are less familiar, walk us through the Airbyte basics at that point. And then maybe just tell us what you started seeing in the market and how you navigated participating in the newly formed ecosystem?
Yeah, so the value prop of Airbyte is very simple. You have data somewhere, and Airbyte gives you an ability to bring it into a place where you will be able to make something out of it and derive value. And at the time we were very focused on data warehouses because every single company was changing their data stack. Now when ChatGPT started to really blow up, we started to go to some of these AI meetups. And we started to look at adjacent open source projects. At the time, Langchain had barely started, but we saw that they were building data connectors.
They were building ways to connect to systems and to remove complexity from building these LLM applications. That’s when we decided – let's go to some meetups, let's do some presentations, let's talk about Airbyte. Let's use the terminology that we use in the data world in a context where the audience is mostly AI engineers or people who work in AI. The concept of ETL did not exist in AI, so we had to rethink how we were talking about data movement, because the wheels were clearly being reinvented here in terms of data movement.
So at that point, we said, "Okay, can we do something to just give more power to people so that they focus on building their chain, building their prompts, building their AI apps, so they don't focus on the data movement piece?" That's really what triggered us to start to work on these very AI-specific connectors, like unstructured sources and vector databases.
I'm going to jump around and break the flow a little bit because I think this is a very natural way to go. We talked about this a year ago, when all of this started happening, and what really stuck out to me was you and the rest of the team really viewed all the AI workloads as just another sort of source-sync combination for Airbyte. And I think a fun thing to talk through is what do you view as the similarities and differences between the "traditional" and the AI-native data stack? What changes about the transformation layer?
It's all a layering of infrastructure. It starts at the lowest level, which is storage. On top of that, you have compute, like GPUs. On top of that, you have data, and on top of that you have AI applications. And the question is, how do you create a solid pyramid? Yeah, people are GPU-poor these days because nobody is able to get compute, but this is critical because it's a piece of building an AI infrastructure, but data is also a critical piece to building infrastructure. You need to create a bridge between the data and the AI world. Before it was more the data and the analytics world, the data and the activation world, but now you need to create a bridge for AI.
So what are these bridges that need to be created? What are the paradigms that are changing and how are they changing? We went from ETL to ELT, and now what's new for AI? When you start dealing with unstructured sources, there is a world in which the T goes back between the E and the L. That's basically what's happening. Maybe you need to start transforming your data a little bit earlier and inject your business logic a little bit earlier before you push it into your publication stores.
At Airbyte, we've built these fundamentals in such a way that we can create abstractions on where the transformation is happening. Today, it's mostly in the warehouse, but we're starting to also investigate how we bring in more logic while data is flowing. So overall, the concepts are the same. It's just reordering letters, one way or another, and you inject business logic. For example, how do you understand the PDF? Well, how do you chunk a PDF? Well, that's your business logic. How do you make sure that it's chunked in the way that’s the most retrievable?
ETLT!
So, very good. But it’s not exactly what we want to call it. We have a joke because we like putting letters together. We call it EMLTP. Okay, now let me explain each of these letters, but that's a joke, to be clear.
So E is the way of extracting data from a source. M is – for people that are familiar with MapReduce – is just mapping your data, so applying simple transformation at the record level, and that could be chunking, it could be about how you interpret an image and transform it into data that can be understood downstream. Then you have the L, which is about loading that data into another place where you can start doing more advanced processing, T.
Lastly you have the P, which is how you publish that data. Once it's transformed, you need to make that data available into stores where it will be usable. So if you're using a vector store, that can be your destination where the data is being published. It's not your raw data, it's not your transformed data. It's data that has been embedded and you've been able to index it in a very specific way that can be retrieved by your AI apps.
You guys had a couple of big product releases a couple of weeks ago, a Pinecone destination being one of them. Maybe to make it a bit less abstract, what are the types of things you guys are seeing being built on top of the Airbyte connectors in the new AI-native world?
We did not have unstructured sources earlier – that's something that the team is actively working on, so expect to see more about that. We focus very much on extracting unstructured data out of APIs.
So where do companies have unstructured data? They have unstructured data on their chat system, like Zendesk. They have unstructured data on Salesforce. The use case we are seeing is people extracting raw data that is being generated by business users, bringing it somewhere, and extracting insight. The main data use case we see is structuring that data. So if you have a chat log, can you extract sentiment? Can you extract features? Ask where the product is failing?
I think it's pretty clear now we're at a place where the rest of the ecosystem is slowly coming around to the fact that getting the right set of data inputs into these systems is sort of a prerequisite to doing anything else. As a first order effect of that, there's a lot of different attempts at this from different corners of the market, whether it be open source frameworks, established players like you, net new unstructured ETL companies, etc.
You're a CEO of the business. You want to be indexed to high growth areas that are taking off. There's a lot of noise around you, and being an active participant of the ecosystem is very important. How do you navigate that? How do you decide where to go after things head on, where to partner with people? How does the business navigate that?
Yeah. I think it's just that we know what the value prop is of the product we have. So for us, it's just a matter of converting the problems we're seeing into the business value that we provide. And if the value prop of Airbyte is that we can move data from point A to point B simply, then every time data is siloed somewhere and needs to go somewhere else, we need to find a way to get it there.
I think we can draw a pretty nice parallel with GPUs, for example. Nvidia built GPUs for games. And then, GPUs were being used for mining Bitcoins, and now GPUs are being used for AI. It's exactly the same thing – the moment you have a platform and you know the capability and the value prop of your platform, then you can position your product in a way that is very clear about how it helps a new industry or new wave of technology.
The other question as it relates to the rest of the ecosystem is, how do you see this playing out? Particularly with data infrastructure for AI.
Yeah. Data is just the gold of every company. I think we've barely touched the surface of how we can leverage large language models on top of unstructured data. If you look at unstructured data, you have two types. You have just raw text data, but you also have other types of data modalities like images. Now, this data is the biggest piece of data that companies own. I don't know if my number is correct, but I will estimate that to be at least 80% of what every single company owns as data. And this is untapped data because it was capital-inefficient.
If you want to do something and structure that unstructured data, you need to start a team to annotate your data, to start interpreting your data, to exercise some judgment that might not be auditable on top of that data. And I think what I see coming in the next few years is more use cases tapping into that data because we have barely scratched the surface. I think today people are barely focusing on – “Let's do this on a few Google Docs or a few PDFs or a few research papers,” but this is just the tip of the iceberg. The data space is going to continue to evolve alongside new use cases.