8VC’s Investment in Unlearn.AI
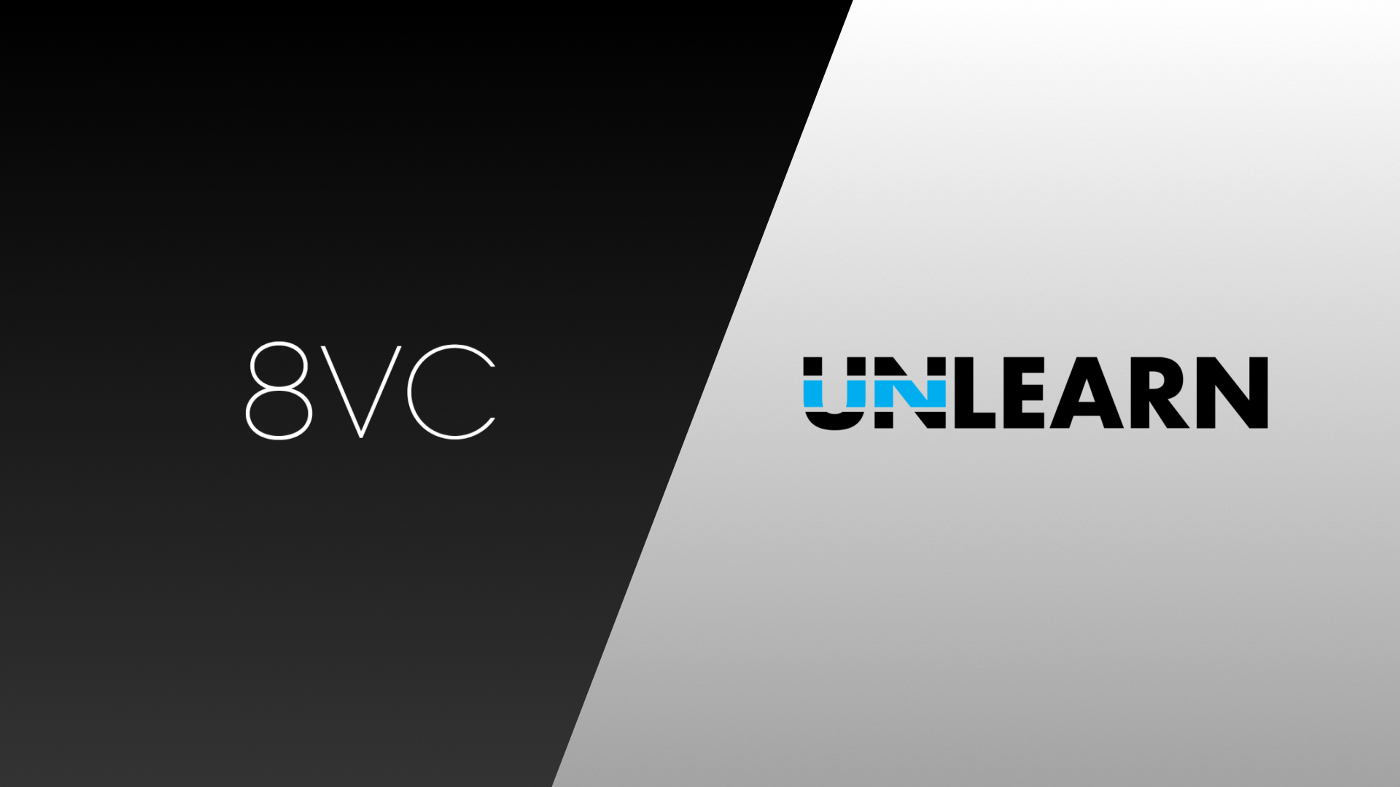
Share
Imagine you have an incurable disease — maybe Alzheimer’s or multiple sclerosis. Though you might be willing to take a chance on science, you would nonetheless face a difficult decision in choosing whether to volunteer for a clinical trial. While you could potentially receive a better treatment, it’s equally likely that you would instead receive a placebo as part of the control arm of the study, used to verify the efficacy of the experimental drug. Despite bravely seeking to improve your life, you might end up as just a baseline comparator. That system doesn’t seem right.
What if, instead, there was a 100% chance you’d get the new therapy, because the trial didn’t need a placebo arm?
Unlearn.AI is changing how we treat patients with medicines in trials. Their invention of “digital twins” allows drugs to be tested in randomized controlled trials (RCTs) without the ethical and financial costs of the placebo control. 8VC is excited to announce that we are leading a $12M Series A investment in Unlearn to help them revolutionize RCTs.
Placebo arms: a necessarily evil
The most problematic element of clinical trials is the placebo control. Since the adoption of the RCT as the gold standard of clinical trial design, the ethics of giving patients “sham” treatments has been hotly debated. The prevailing philosophy has been that the harm inflicted by giving patients a placebo is justified, because even though it means denying those patients a real treatment, it’s still less bad than the possibility of approving a costly new drug that might not actually help and could possibly even be harmful.
A quick look at the numbers is sobering. Right now, there are roughly 700 placebo-controlled, phase III clinical trials actively recruiting in the US. On average, about 60% are expected to reach approval. Those figures indicate that we’re denying efficacious therapies to hundreds of thousands of people in this current batch of trials alone. In a system where joining a trial means rolling the dice on getting a real treatment or a sham, it’s no surprise that enrollment challenges lead to such a high proportion of trials blowing past their budgets and timelines. Many studies fall short of target patient numbers altogether.
How Unlearn is changing the math
Unlearn’s approach to the ethical quandary of placebos takes inspiration from the idea of “twin studies,” where scientists evaluate treatment effects with identical twins, giving a treatment to one twin and leaving the other twin untreated as a control. This paradigm allows researchers to control for genetic variation since identical twins share the same genome.
The question the Unlearn founders asked was “can we create a virtual version of a twin for any treated patient, to avoid needing a placebo arm?” Drawing upon his experience in biostatistics at Pfizer and his background as a research biophysicist, Unlearn CEO Charles Fisher has been leading the company to the solution. Unlearn builds computational models trained on data from prior placebo patients for a given indication. Using these models, Unlearn generates virtual control patients with features indistinguishable from real ones. They call the virtual patients “digital twins.”
The core statistical approach for correctly modeling the patients is called a Boltzmann-Encoded Adversarial Machine (BEAM) and was recently invented by Unlearn’s team, a group of top scientists and engineers with PhDs from elite institutions such as Harvard, UPenn, Stanford, McGill, and UW. BEAMs handle the myriad particulars of patient data, including missingness, time-series components, and mixtures of discrete and continuous data types, all with the placebo effects of the training data automatically built in. When the BEAM is seeded with new patient data at the start of a trial, it generates digital twins for every patient, predicting how they would trend if they were to be assigned to the placebo arm, and yielding outputs that are statistically indistinguishable from the biomarkers of real placebo patients.
For each indication, placebo data from prior trials is used to train a BEAM that captures the indication’s unique trajectories and how those relate to the relevant clinical endpoint. Pharma, researchers, and patient advocacy organizations are all aligned and highly motivated to reduce or remove placebo arms from trials. Unlearn has partnered with numerous groups to amass the placebo data needed for model training, in pursuit of a set of models that would completely cover the hundreds of placebo-controlled trials starting every single year. Through their team’s considerable work in data cleaning and integration, they’ve already successfully trained BEAMs for several indications.
Putting history in the past
A related initiative is the development of the “synthetic control arm” (SCA) to replace the placebo arm. In trials with an SCA, the treated group is compared to a prior group of patients, either placebo arms from historical trials or real-world evidence from historical patients’ healthcare records. The SCA has seen significant adoption in oncology, where placebo assignment is often untenable due to the risk of mortality, and thus the drug approval processes are sometimes given more leeway in the use of single-arm trial designs. Several multi-billion-dollar companies have been built around collating and selling SCA data in this context.
However, SCA trials have limited statistical power and introduce bias. Whereas Unlearn uses advanced computational techniques to generate nearly identical digital twins for every patient, SCAs use statistical methods from the early twentieth century to match the current patients to a subset of the prior ones based on a set of preselected features. The historical placebo data set for an SCA needs to be massive in order to have sufficient breadth to provide good statistical matches for the treated population, and such large cohorts of placebo patients usually do not exist, particularly for orphan diseases. Because there is rarely any subset of historical placebo patients that can form a perfect match for the current patients, SCA trials settle for an improperly controlled “control” group.
For these reasons, prominent researchers such as Judea Pearl have criticized the statistical matching methods that form the basis of SCA, noting that choosing the wrong set of preselected features to match on can actually increase the bias of a study. These types of trials have generated controversy in the past for facilitating FDA approval of drugs that ultimately show little patient benefit. The main argument for their continued use has simply been that for an oncology trial without a placebo arm, including historical data is probably better than completely omitting the control. Because Unlearn’s approach does not suffer from these same statistical issues, they will make SCA’s problematic use of historical data truly a thing of the past.
A future of better care, at lower costs
The promise of Unlearn extends far beyond the direct ethical benefits of subjecting fewer patients to sham treatments. In our diligence, we repeatedly saw how their solution can radically improve patient care while substantially reducing costs.
Medical researchers have promised precision medicine for decades. It has even been an official matter of US policy since President Obama’s 2015 State of the Union address. Yet, precision medicine has been slow to deliver. Our drug approval processes have largely been stagnant in their ability to evaluate whether new treatments are expected to be effective at the level of individual patients. Unlearn offers a natural solution to this problem. Because Unlearn can simulate multiple digital twins per patient, covering the range of probable paths a patient might follow, their approach allows for the calculation of per-patient p-values in trials.
Unlearn will also massively reduce the cost of clinical trials. Recruiting, treating, monitoring, and evaluating patients requires highly specialized healthcare — with pharma paying at least as much as healthcare insurance companies do. By reducing placebo populations, Unlearn can therefore cut up to half of clinical trial costs, which represent the most expensive component of drug development and which bleed over into the drug pricing that patients and our healthcare system bear. Reducing recruitment of hundreds of patients represents tens of millions in savings in development of approved therapies. When deployed in trials at the upper end of enrollment targets, the cost reduction can be in excess of a hundred million dollars for a single drug.
Equally important, lower enrollment targets increase trial speed. Unlearn thus delivers further savings, along with substantial increases in total drug revenues, by shortening phase II and III trials from their current combined timeframe of roughly five years. Their approach allows trials to proceed at twice the normal speed, simultaneously enabling drugs to be retailed at lower prices once they receive approval. Because Unlearn delivers such enormous business value, they are poised to be a massive player in the clinical trials ecosystem.
In placebo-controlled trials there are no perfect choices, and until now society has been forced to normalize the near-term harm of a placebo arm so we can avoid the long-term harm of letting potentially ineffective drugs come to market. Now that Unlearn has brought the relevant data sets and methods into place, they are moving the field beyond the eighteenth-century idea of the placebo and the statistical matching approaches of the early twentieth century, to drive forward a patient care paradigm befitting the twenty-first century.
How you can help realize this vision
We are thrilled to partner with Unlearn. If you’re excited, too, there are several ways to help.
Hiring: Unlearn is building out the world’s strongest clinical AI team to solve deep technical problems with complex data sets. Would you or somebody you know want to help tackle the challenges?
Data: The more historical patient data we have, the more indication-specific models Unlearn can build. Do you have connections to clinical trial data consortia that might be helpful here?
Partnerships: Unlearn is focused on proving that their techniques work across many different studies. Are you running a clinical trial that might benefit from their approach?
Championing: Unlearn is changing the paradigm of clinical trials. We need to bring their approach to the forefront of the medical research conversation. The more people involved, the more we can improve patient care.